The report considers the four major types of attacks: evasion, poisoning, privacy and abuse attacks. It also classifies them according to multiple criteria such as the attacker's goals and objectives, capabilities, and knowledge. Evasion attacks, which occur after an AI system is deployed, attempt to alter an input to change how the system. So data pooling means that you can: combine together data on one individual coming from multiple sources such as medical devices, specialist clinics, health records. merge into one file multiple datasets from many patients coming from various countries or institutions.

Hasil Pengeluaran Togel Petaling Hari Ini Selasa, 17 November 2020
Data pooling is basically what it sounds like - combining together data to improve the overall effectiveness. This is otherwise known as second party data. Given the need to develop better customer relationships, companies are now looking beyond their own customer data to create a more well-rounded view. The pool is developed as a stand alone pool to meet the needs of a specific deliverable The pool will be periodically updated (appended to) and re-run - e.g. to support DSURs It is common for a project to develop a number of data pools to support a variety of activities that require pooled analysis. Data pooling is basically what it sounds like - combining together data to improve the overall effectiveness. This is otherwise known as second party data. Given the need to develop better customer relationships, companies are now looking beyond their own customer data to create a more well-rounded view. Data Pooling in Capital Markets and its Implications March 15, 2020 by MAIEI In this guest post, Jimmy Huang (Subject Matter Expert for Data Pooling at TickSmith) explains the origin story of data pooling in the banking sector, and its ethical implications in an increasingly AI-driven world.

Le Meridien Petaling Jaya opens its door for guests Penang Hyperlocal
A data pool is a collection of data created for the purpose of analysis. It can be large or small, and the methods used to collect the data can influence the accuracy of the values within the pool. Manual data collection is usually reliable, but automatic data collection provides the best accuracy. In practice, we find that direct pooling of already collected datasets in a post hoc manner across multiple sites can be problematic due to differences in the distributions of one or more measures (or features) ().In fact, even when data acquisition is harmonized across sites, we may still need to deal with site-specific or method-specific effects on the measurements, such as the above noted. Creating profiles from data pools for personalised service and marketing. The combination of databases and datasets across separate entities in a group of companies or across different business segments can very often lead to greatly improved insights into how the group's products and services are used. These insights, gained through a deep. This intuition further suggests that data-pooling offers the most benefits when there are many problems, each of which has a small amount of relevant data. Finally, we demonstrate the practical benefits of data-pooling using real data from a chain of retail drug stores in the context of inventory management.

Sapphire Paradigm Petaling Jaya
18 This question does not show any research effort; it is unclear or not useful Save this question. Show activity on this post. I thought that 'pooling data' simply meant combining data that was previously split into categories.essentially, ignoring the categories and making the data set one giant 'pool' of data. In the Internet of Things, data pooling allows manufacturers to have a data allowance for all their devices, rather than paying per device and needing to pay additionally for devices that exceed the data per device. At emnify, you only have to pay for active IoT SIM cards. It's the secret to our usage-based pricing model.
Data pooling is a process where data sets coming from different sources are combined. This can mean two things. First, that multiple datasets containing information on many patients from different countries or from different institutions is merged into one data file. Second, that data on one patient, coming from multiple sources such as e.g. With Pools, Databricks customers eliminate slow cluster start and auto-scaling times. Data Engineers can reduce the time it takes to run short jobs in their data pipeline, thereby providing better SLAs to their downstream teams. Data Analytics teams can scale out clusters faster to decrease query execution time, increasing the recency of.
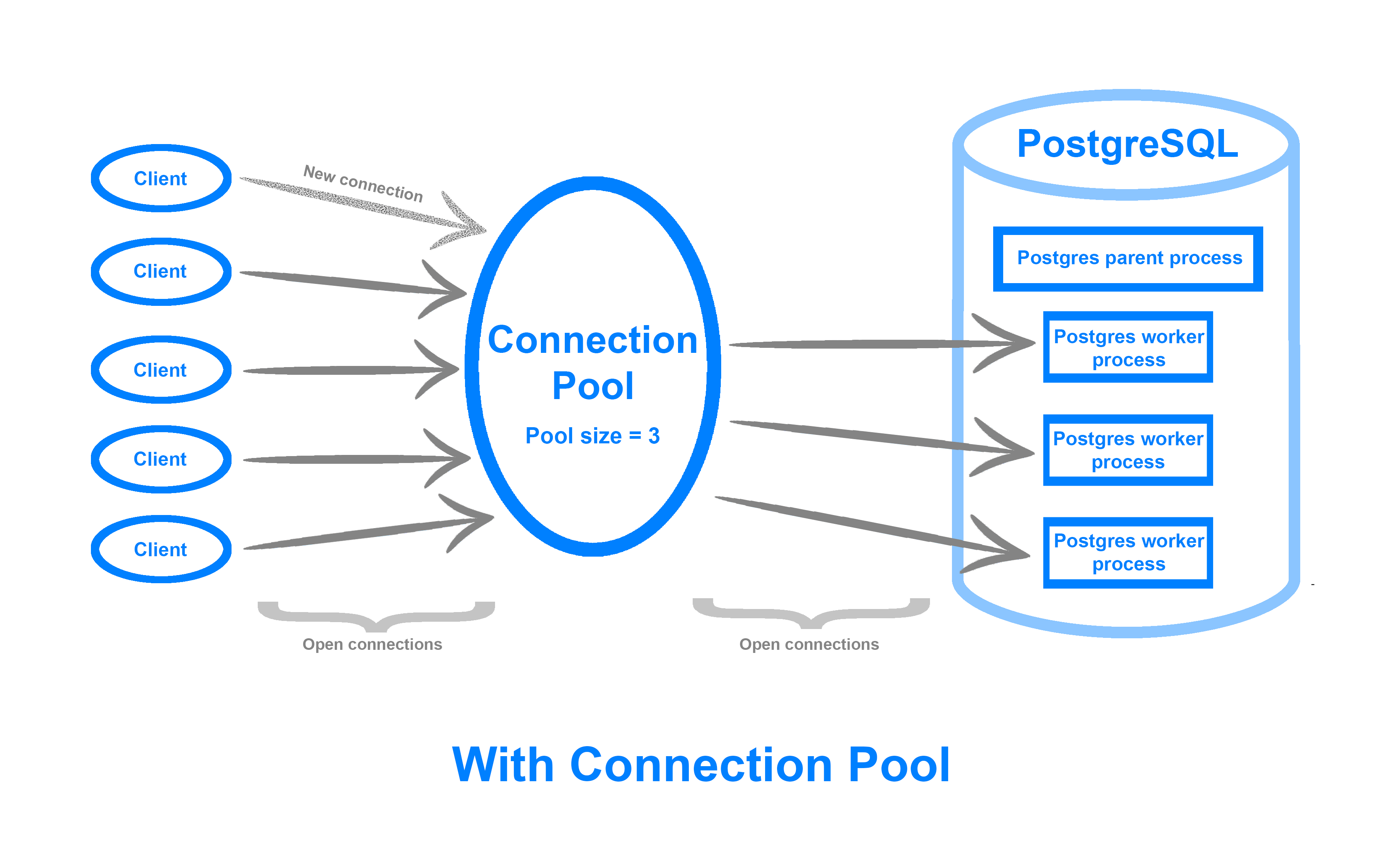
Managed Databases Connection Pools and PostgreSQL Benchmarking Using
A data pool consists of a Kubernetes cluster that facilitates managing multiple data pool projects. Each data pool runs independently, and budget and resources are allocated considering the individual project's demands. Thus, costs are more predictable per project. So, 'pooling the data' means combining all of the data points from various sites into a single large collection so that you can run detection functions on the entire collection and calculate a density estimate, where if you had run the detection on a single site's data set, there would not have been enough data in the set to make a decent estimate.