Thomas van Aquinostraat 4. 6525 GD NIJMEGEN. Postal address. Postbus 9104. 6500 HE NIJMEGEN. Working days Monday, Tuesday, Wednesday, Thursday, Friday. About. Marcel van Gerven focuses on the development of artificial intelligence (AI) that is inspired by the way in which the human brain works. This provides the basis for smarter and more. Marcel van Gerven. Professor of Artificial Cognitive Systems, Donders Institute for Brain, Cognition and Behaviour. Verified email at donders.ru.nl. MAJ van Gerven, K Friston. Scientific reports 7 (1), 5677, 2017. 214: 2017: Attention modulations of posterior alpha as a control signal for two-dimensional brain-computer interfaces. M Van.
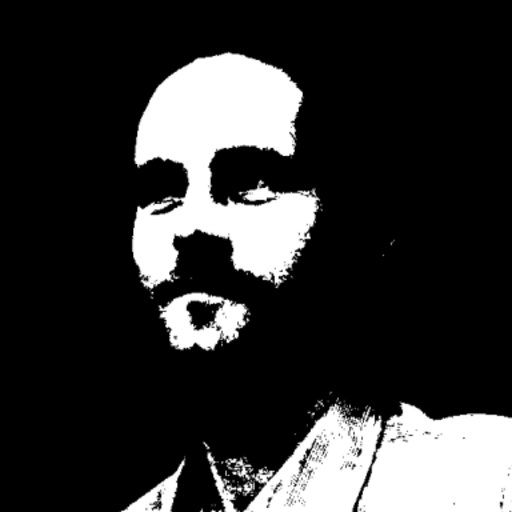
Marcel VAN GERVEN PhD Radboud University, Nijmegen RU Donders Institute for Brain
Marcel van Gerven Segmenting perceptual experience into meaningful events is a key cognitive process that helps us make sense of what is happening around us in the moment, as well as helping us. Marcel van Gerven is Professor of Artificial Cognitive Systems and Principal Investigator in the Donders Institute for Brain, Cognition and Behaviour. He received his MSc Degree in Cognitive Science in 2000 and his PhD in Medical Informatics in 2007 from Radboud University. Van Gerven is chair of the AI department at Radboud University. Marcel van Gerven is a professor of Artificial Cognitive Systems and chair of the Department of Artificial Intelligence at the Donders Institute for Brain, Cognition and Behaviour at Radboud University. He is recipient of an NWO Vidi grant. His lab focuses on understanding the computational basis of cognitive processes and the development of. Radboud Universiteit Donders Institute for Brain Cognition and Behaviour: Nijmegen, Gelderland, NL
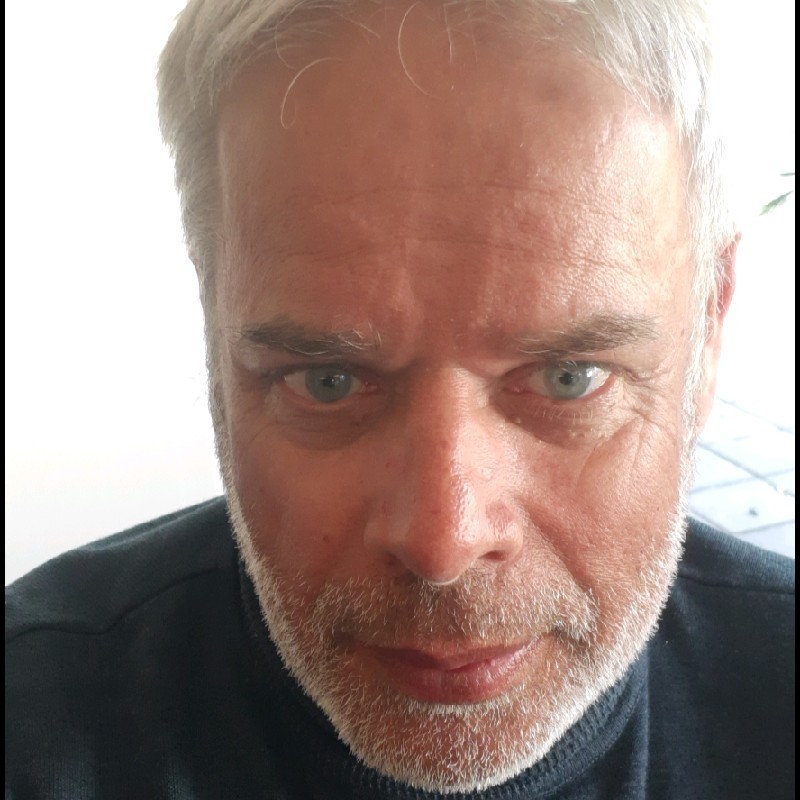
Marcel van Gerven LinkedIn
Marcel van Gerven According to most theories, perceptual switching during binocular rivalry is caused by competition between the neural representations of the two input images. Marcel van Gerven 1 Affiliation 1 Computational Cognitive Neuroscience Lab, Department of Artificial Intelligence, Donders Institute for Brain, Cognition and Behaviour, Radboud University, Nijmegen, Netherlands. PMID: 29375355 PMCID: PMC5770642 DOI: 10.3389/fncom.2017.00112. Marcel A. J. van Gerven's 75 research works with 1,278 citations and 19,278 reads, including: Predictive coding is a consequence of energy efficiency in recurrent neural networks Marcel van Gerven, Radboud University Nijmegen, Donders Institute for Brain, Cognition and Behaviour, Faculty Member. Studies Machine Learning, Cognitive Neuroscience, and Neuroinformatics.
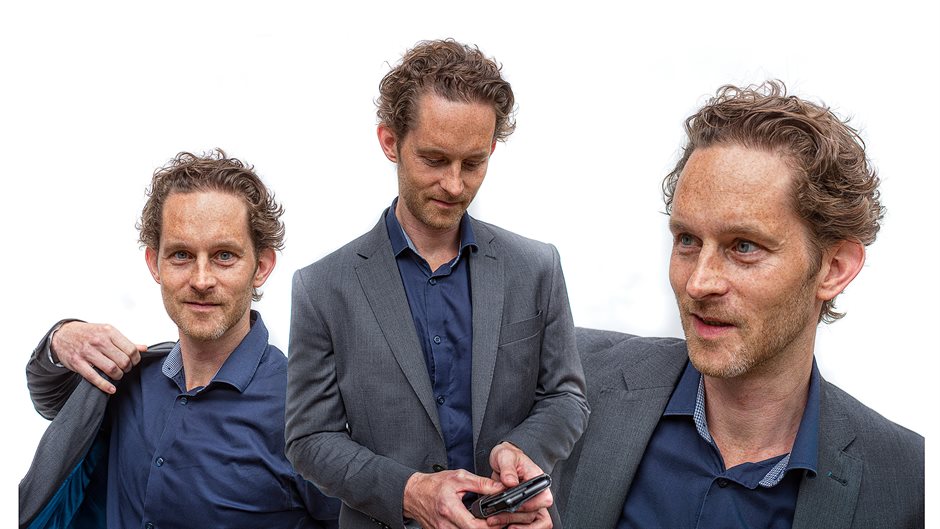
‘Als we echt intelligente machines willen bouwen dan moeten we de mens omarmen’ Emerce
Marcel van Gerven 1 , Sander Bohte 2 Affiliations 1 Department of Artificial Intelligence, Donders Institute for Brain, Cognition and Behaviour, Radboud University Nijmegen, Nijmegen, Netherlands. 2 Department of Machine Learning, Centrum Wiskunde and Informatica, Amsterdam, Netherlands. PMID: 29311884 PMCID. Marcel van Gerven. Radboud University Nijmegen, Nijmegen, The Netherlands. View editor publications. You can also search for this editor in PubMed Google Scholar. Presents a snapshot of explainable and interpretable models in the context of computer vision and machine learning. Covers fundamental topics to serve as a reference for newcomers to.
Explainable Deep Learning: A Field Guide for the Uninitiated. Gabrielle Ras, Ning Xie, Marcel van Gerven, Derek Doran. Deep neural networks (DNNs) have become a proven and indispensable machine learning tool. As a black-box model, it remains difficult to diagnose what aspects of the model's input drive the decisions of a DNN. Bodo Rueckauer 1 , Marcel van Gerven 1 Affiliation 1 Department of Artificial Intelligence, Donders Institute for Brain, Cognition and Behavior, Radboud University, Nijmegen, Netherlands. PMID: 36968496 PMCID: PMC10030734 DOI: 10.3389/fnins.2023.1141884.
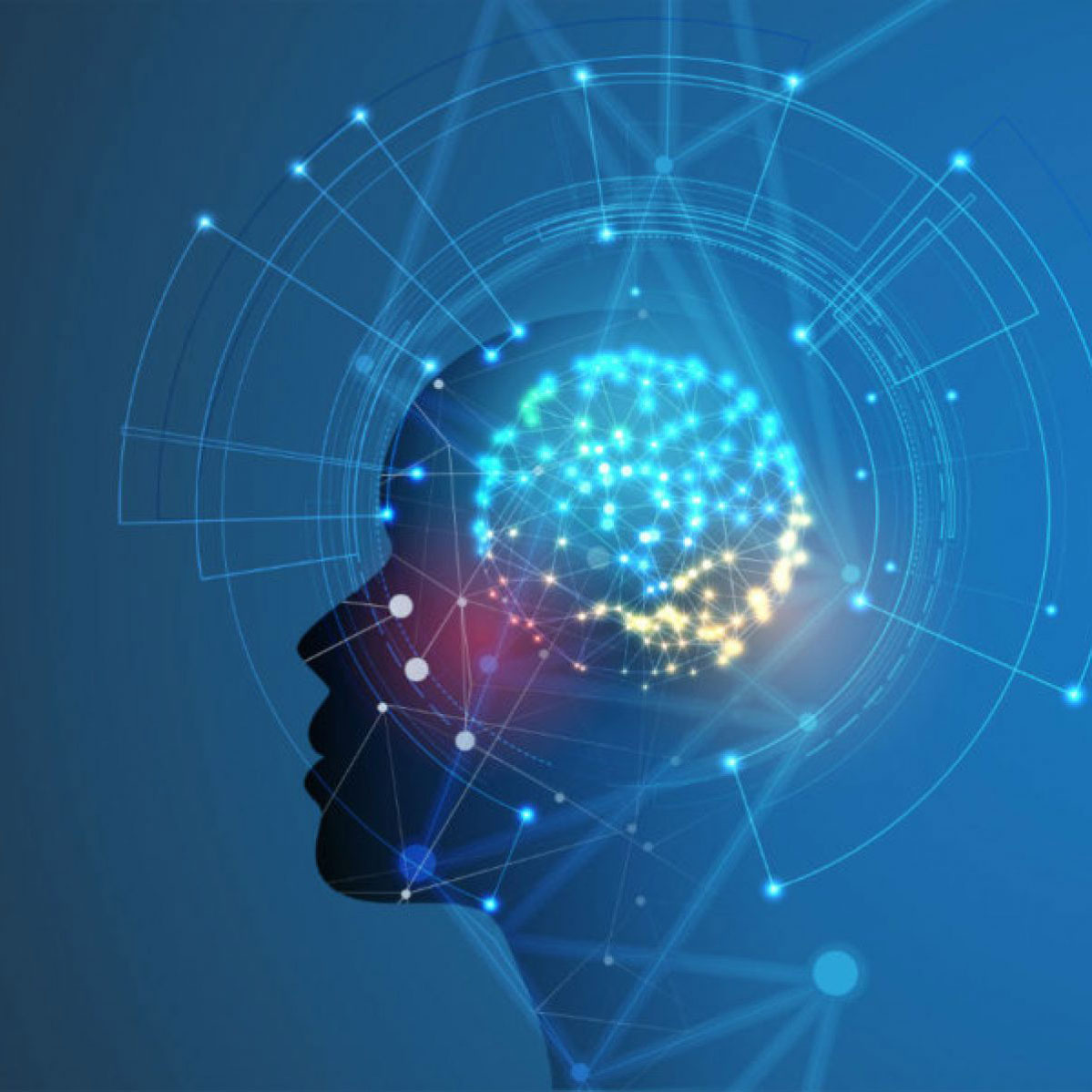
Intense Project Marcel van Gerven
Marcel van Gerven Principal Investigator-Donders Institute. I am interested in the theoretical and computational principles that allow the brain to generate optimal behavior based on sparse reward signals provided by the environment. We create biologically plausible neural network models that further our understanding of natural intelligence. Stochastic variational inference offers an attractive option as a default method for differentiable probabilistic programming. However, the performance of the variational approach depends on the choice of an appropriate variational family. Here, we introduce automatic structured variational inference (ASVI), a fully automated method for constructing structured variational families, inspired by.