Amazon SageMaker automatic model tuning (AMT), also known as hyperparameter tuning, finds the best version of a model by running many training jobs on your dataset. To do this, AMT uses the algorithm and ranges of hyperparameters that you specify. AutoML allows you to derive rapid, general insights from your data right at the beginning of a machine learning (ML) project lifecycle. Understanding up front which preprocessing techniques and algorithm types provide best results reduces the time to develop, train, and deploy the right model.
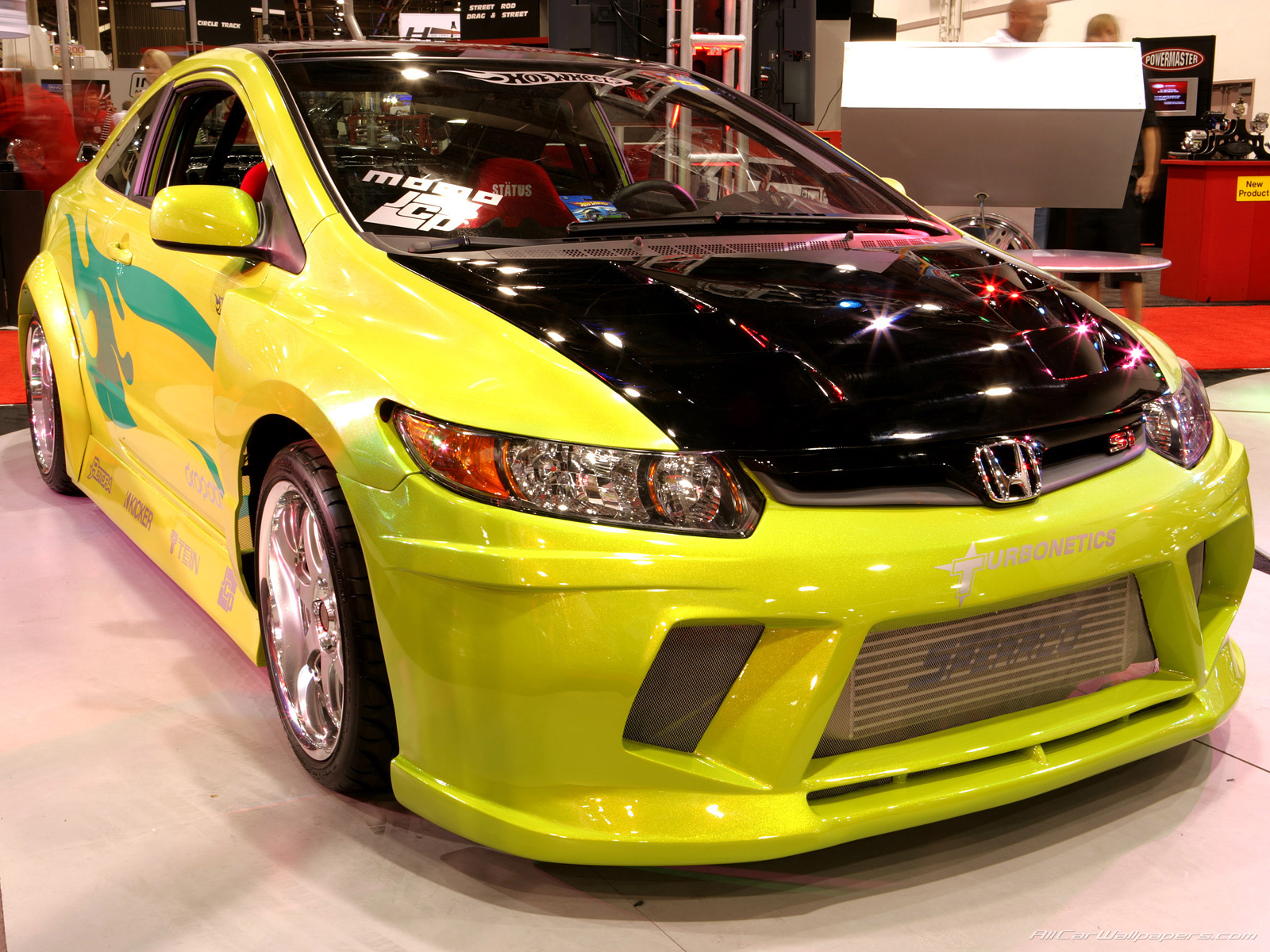
Autos Autos Tuning
Solution overview This technical workflow gives an overview of the different Amazon Sagemaker features and steps needed to automatically tune a JumpStart model. In the following sections, we provide a step-by-step walkthrough of how to run automatic model tuning with JumpStart using the LightGBM algorithm. Automatic Model Tuning eliminates the undifferentiated heavy lifting required to search the hyperparameter space for more accurate models. This feature allows developers and data scientists to save significant time and effort in training and tuning their machine learning models. Amazon SageMaker Automatic Model Tuning has introduced Autotune, a new feature to automatically choose hyperparameters on your behalf. This provides an accelerated and more efficient way to find hyperparameter ranges, and can provide significant optimized budget and time management for your automatic model tuning jobs. For an example notebook that uses random search, see the Random search and hyperparameter scaling with SageMaker XGBoost and Automatic Model Tuning notebook. Bayesian Optimization. Bayesian optimization treats hyperparameter tuning like a regression problem. Given a set of input features (the hyperparameters), hyperparameter tuning optimizes a.
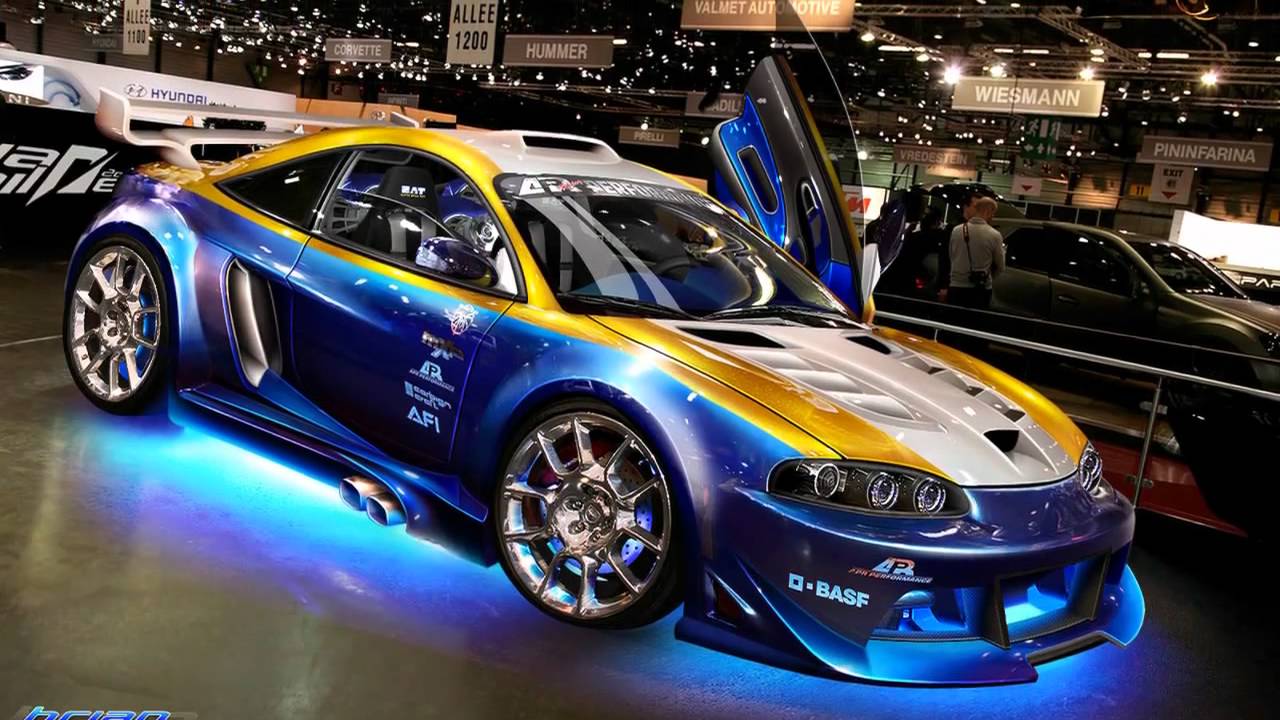
Auto Tuning 2012 YouTube
Amazon SageMaker Automatic Model Tuning As an ML practitioner using SageMaker AMT, you can focus on the following: Providing a training job Defining the right objective metric matching your task Scoping the hyperparameter search space Automatic model tuning, also known as hyperparameter tuning, finds the best version of a model by running many jobs that test a range of hyperparameters on your dataset. You choose the tunable hyperparameters, a range of values for each, and an objective metric. You choose the objective metric from the metrics that the algorithm computes. the tuning. This paper presents Amazon SageMaker Automatic Model Tuning (AMT), a fully managed system for gradient-free optimization at scale. AMT finds the best version of a trained ma-chine learning model by repeatedly evaluating it with different hyperparameter configurations. It leverages either random search This paper presents Amazon SageMaker Automatic Model Tuning (AMT), a fully managed system for gradient-free optimization at scale. AMT finds the best version of a trained machine learning model by repeatedly evaluating it with different hyperparameter configurations.

Checkout my tuning Tesla ModelS 2013 at 3DTuning 3dtuning tuning
We continue our journey from the post Optimize hyperparameters with Amazon SageMaker Automatic Model Tuning. We previously explored a single job optimization, visualized the outcomes for SageMaker built-in algorithm, and learned about the impact of particular hyperparameter values. Garage CHALLENGES Cars Schedule 3DTuning - Your Ultimate 3D Car Configurator Explore 3DTuning's 3D car configurator. Customize a variety of cars with tuning parts, materials, and suspension settings. Unleash creativity and join our car enthusiasts' community. Cars List AC Cobra Classic Roadster 1962 AC Cobra Classic Roadster 1962
Amazon SageMaker Automatic Model Tuning (AMT) finds the best version of a model by running many SageMaker training jobs on your dataset using the algorithm and ranges of hyperparameters. It then chooses the hyperparameter values that result in a model that performs the best, as measured by a metric (e.g., accuracy, auc, recall) that you define. Amazon SageMaker Developer Guide Best Practices for Hyperparameter Tuning PDF RSS Hyperparameter optimization (HPO) is not a fully-automated process. To improve optimization, follow these best practices for hyperparameter tuning. Topics Choosing a tuning strategy Choosing the number of hyperparameters Choosing hyperparameter ranges
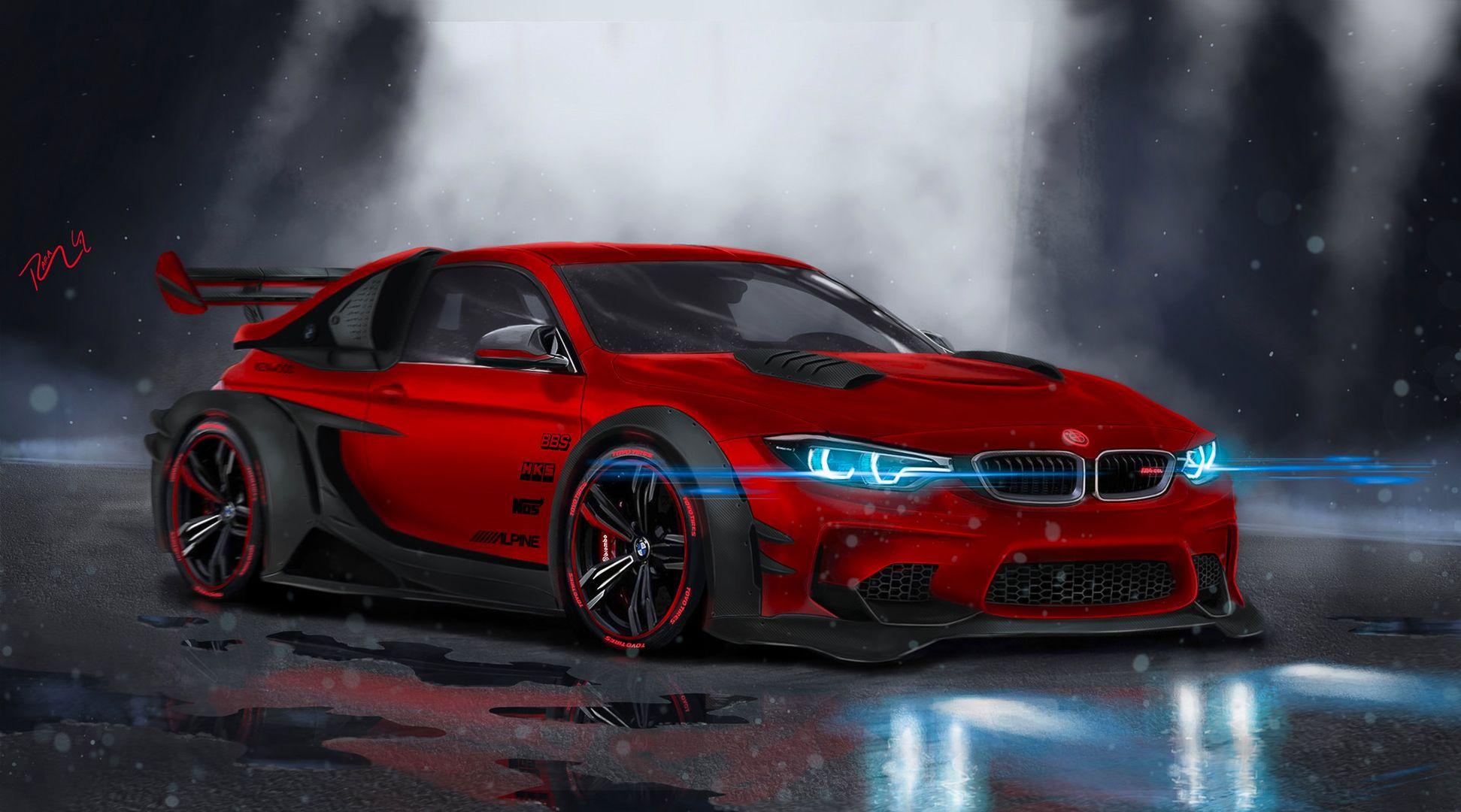
Auto Tuning
Amazon SageMaker's automatic model tuning feature is a game-changer for machine learning practitioners. It not only simplifies the hyperparameter tuning process but also ensures that models. SageMaker Automatic Model Tuning (AMT) may add additional hyperparameters(s) that contribute to the limit of 100 total hyperparameters. Currently, to pass your objective metric to the tuning job for use during training, SageMaker adds _tuning_objective_metric automatically.