1.6.2. Nearest Neighbors Classification¶. Neighbors-based classification is a type of instance-based learning or non-generalizing learning: it does not attempt to construct a general internal model, but simply stores instances of the training data.Classification is computed from a simple majority vote of the nearest neighbors of each point: a query point is assigned the data class which has. Hamming distance. The decomposition is repeated recursively for each cluster until the number of points in a cluster is less than a threshold, in which case this cluster becomes a leaf node. The hierarchical decomposition of the database is repeated for several times and multiple hierarchical trees are constructed. The
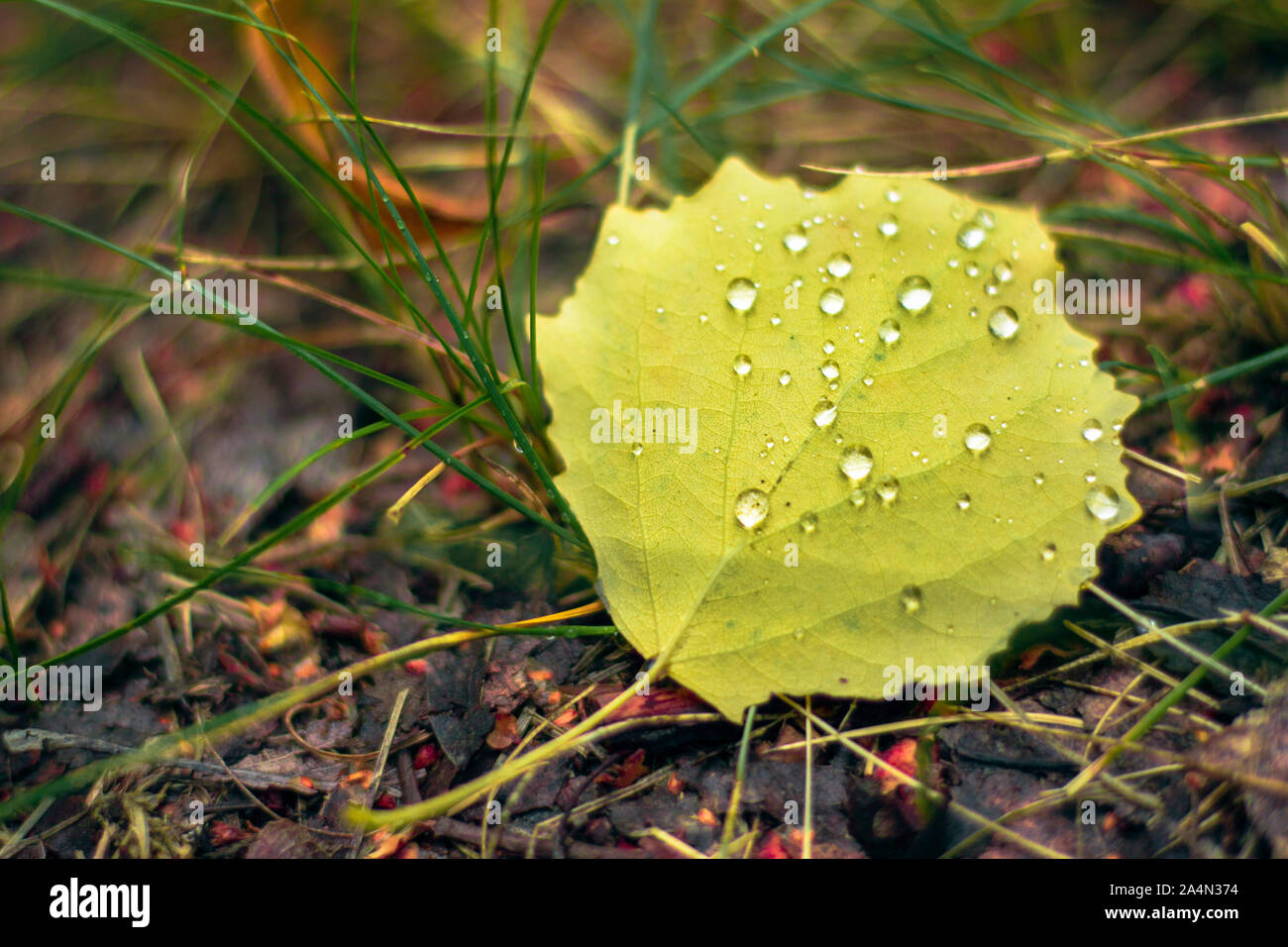
Wet autumn aspen leaf on the undergrowth Stock Photo Alamy
In this Notebook, we will explore a cool new dimensionality reduction technique called Uniform Manifold Approximation and Projection (UMAP) and check its applicability for doing supervised clustering and embedding over the similarity space computed from the leaves of a random forest. Data Leaf size passed to BallTree or KDTree. This can affect the speed of the construction and query, as well as the memory required to store the tree. The optimal value depends on the nature of the problem. p float, default=2. Power parameter for the Minkowski metric. When p = 1, this is equivalent to using manhattan_distance (l1), and euclidean. Tapering (or Weighting) A solution to the sidelobe problem is to apply a weighting across the rectangular pulse. This is common in FFTs, and tapering options in phased arrays are directly analogous to weighting applied in FFTs. The unfortunate drawback of weighting is that sidelobes are reduced at the expense of widening the main lobe. The definition originates from the technological characteristics of next-generation sequencing machines, aiming to link all pairs of distinct reads that have a small Hamming distance or a small shifting offset or both.
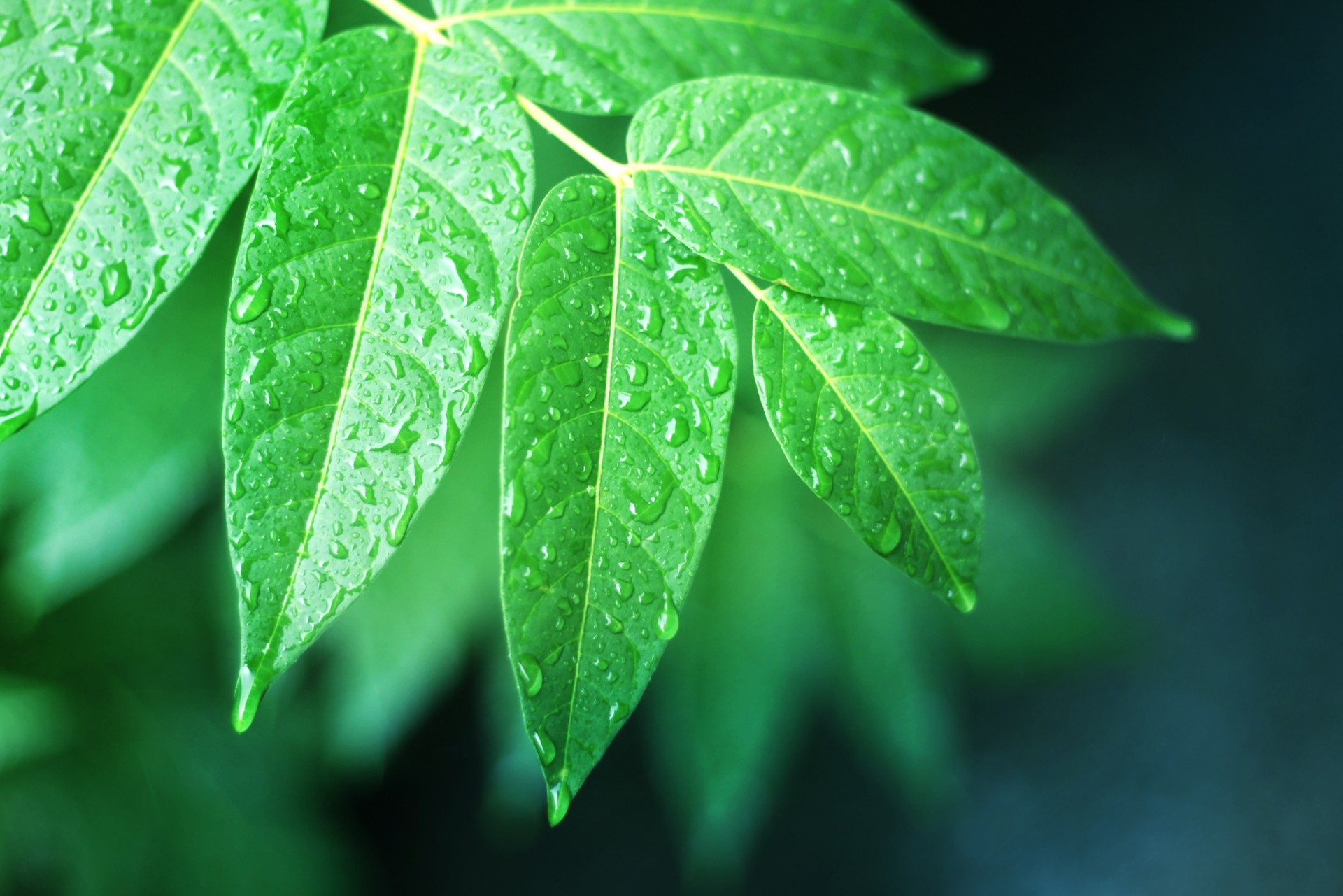
Wet Leaves Free Stock Photo Public Domain Pictures
We address the problem of fast approximate nearest neighbor searching (ANN) in high dimensional Hamming space.. Then, each non-leaf node in the KD-Tree needs 6 bytes to store the splitting dimension (2 bytes are enough for representing dimension as high as 65,536) and threshold value (4 bytes as it is a float value). Go to: Author summary We present a novel graph-based algorithm to compress next-generation short sequencing reads. The novelty of the algorithm is attributed to a new definition of genomic sequence graph named Hamming-Shifting graph. It consists of edges between distinct reads that have a small Hamming distance or a small shifting offset or both. In our case, DNA sequences are taken as the codewords, where L is the number of bases (A,C,G,T) that make up the sequence. The number of positions that two codewords of the same length differ is the Hamming distance. 27 In case of DNA sequences, we define this distance as the number of bases by which they differ. The Hamming graph H(n,m), has Ωn as its vertex-set, with two vertices are adjacent if and only if they differ in exactly one coordinate. In this paper, we provide a proof on the automorphism group of the Hamming graph H(n, m), by using elementary facts of group theory and graph theory. Mathematics Subject Classification (2010).

Yellowing Pecan Nut Leaves Free Stock Photo Public Domain Pictures
From Table 1, we know that References [20, 59] are the most similar studies.Reference [] addressed an exact PM.Reference [] focused on an approximate PM with Hamming distance and proposed an effective algorithm, named SONG, which employed a special designed data structure named single-leaf Nettree to tackle the length and similarity constraints. A Hamming graph H(d,N) is distance-transitive, hence distance-regular. The proofs of the above assertions are straightforward and omitted. The intersection number of a Hamming graph H(d,N) is denoted by pk ij without indicating the parameters d,Nto avoid cumbersome notation. Lemma 5.4. For the Hamming graph H(d,N) we have p0 nn = d n (N−1)n,n.
1. Introduction Traditionally, the two encoding operations of compression and error detection/correction are at odds with one another. Compression techniques reduce redundancy in a set of data. Error correction adds redundant information to a data stream so that errors can be detected and recovered from. Upgrading edges (nodes) can reduce the infectious intensity with contacts by taking prevention measures such as disinfection (treating the confirmed cases, isolating their close contacts or vaccinating the uninfected people). We take the sum of root-leaf distance on a rooted tree as the whole infectious intensity of the tree.
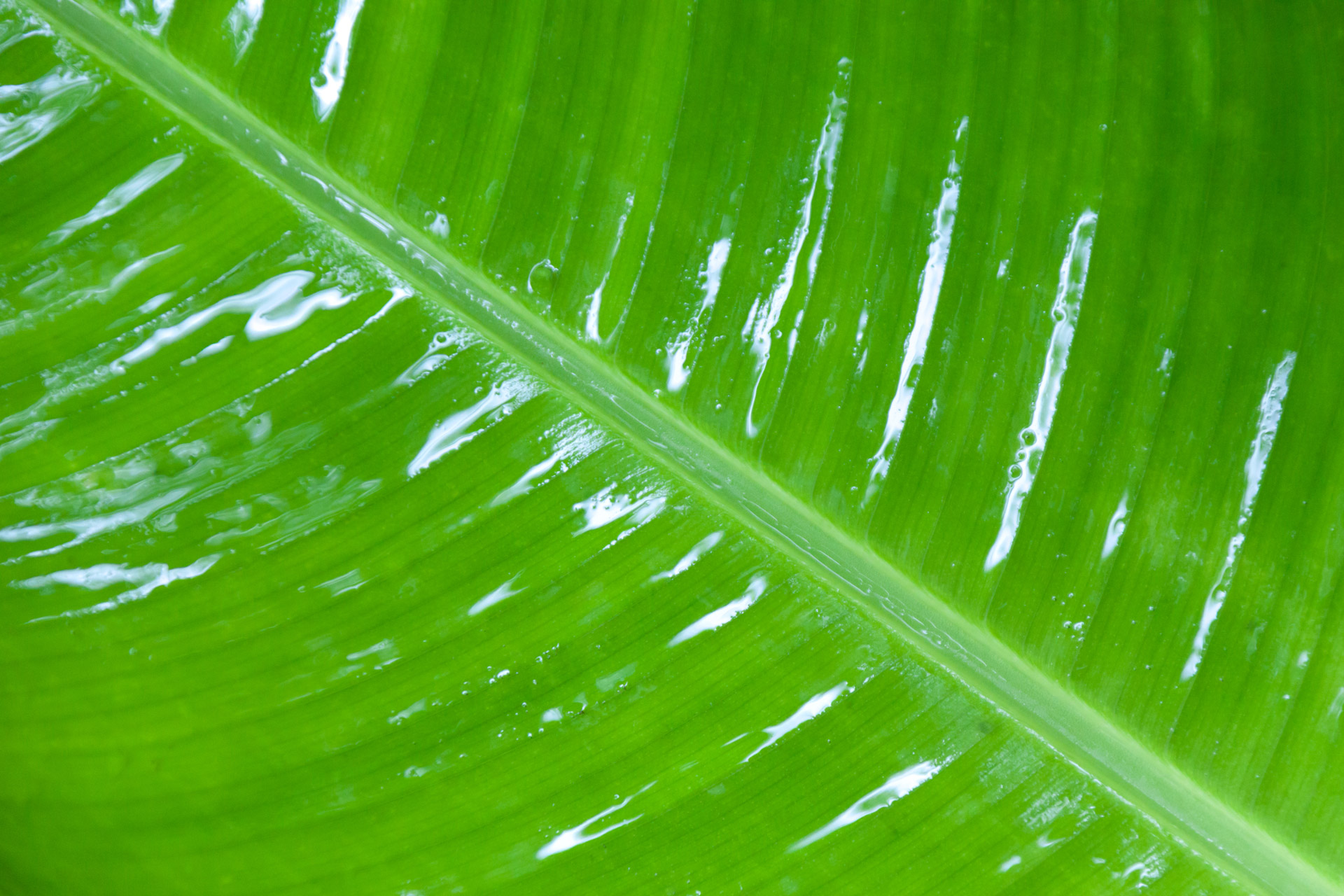
Wet Leaf Detail Free Stock Photo Public Domain Pictures
including Hamming Loss (HL), Subset Accuracy (SA) and Ranking Loss (RL). However, there is a gap between empirical results and the existing theories: 1) an algorithm often empirically performs well on some measure(s) while poorly on others, while a formal theoretical analysis is lacking; and 2) in small label Hamming Leaf. Material obtained from monsters. Used for crafting. ClassID; 645477: ClassName; misc_Haming2